Summary: In today’s episode of The Deep Dive, we delved into the concept of latent thoughts, comparing them to the hidden steps involved in creating a final product, like drawing a cat. These underlying processes play a crucial role in various advancements, from more efficient language models to the development of engaging AI, including in video games. The discussion raised thought-provoking questions about the potential for AI to reason in a more human-like manner. On a personal level, listeners are encouraged to consider how uncovering latent thoughts could enhance their learning and problem-solving abilities. This engaging exploration of hidden understanding offers valuable insights for both tech enthusiasts and individuals seeking to deepen their own understanding. Join us next time for another enlightening deep dive into intriguing topics.
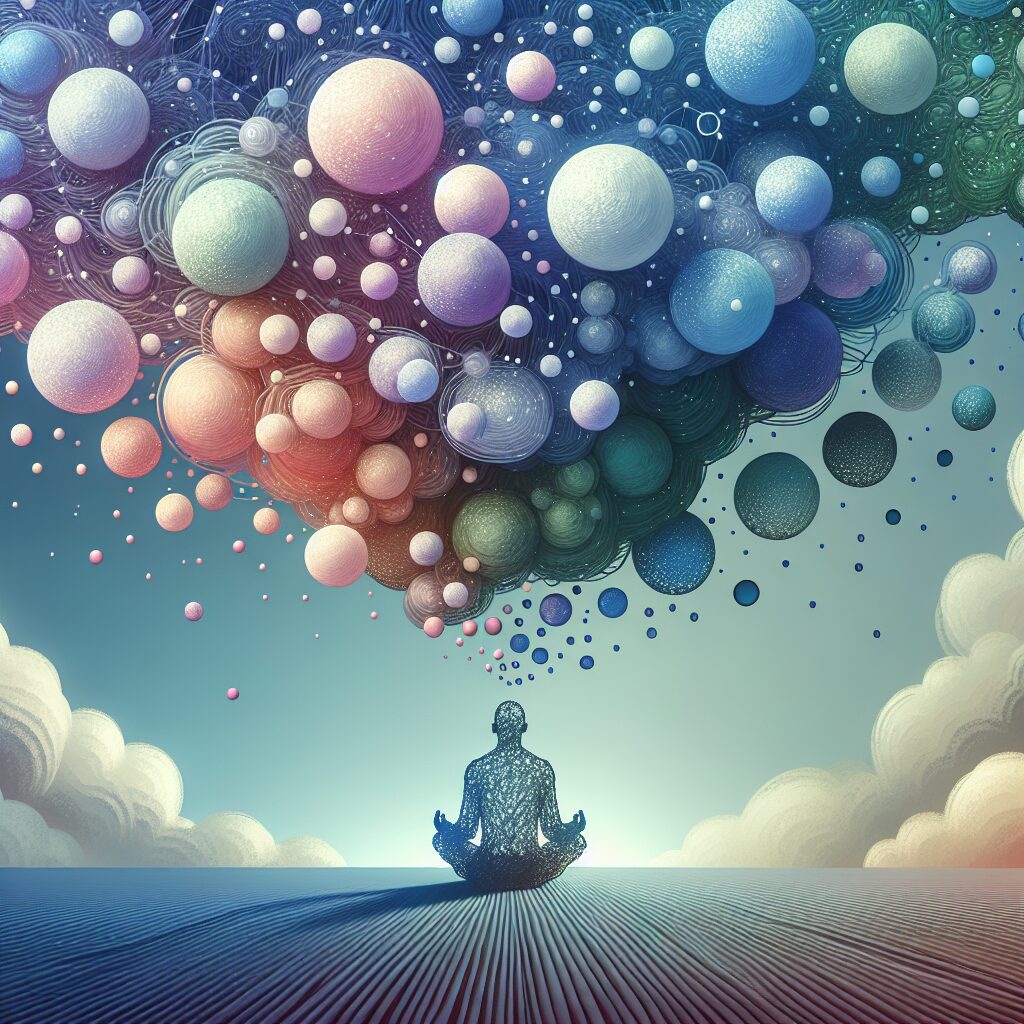
Welcome back to The Deep Dive, where we explore the fascinating realms of technology, artificial intelligence, and the hidden intricacies of human-like reasoning. In today’s episode, we delve into the world of latent thoughts, drawing parallels with the unseen steps involved in creating a masterpiece, like drawing a cat. Just as these hidden processes are crucial to final artistic products, they are equally vital in advancing AI, particularly in language models and video games. Join us as we uncover how understanding these latent thoughts can enhance both machine learning and our own cognitive abilities.
Decoding the Data Bottleneck
In the rapidly evolving world of artificial intelligence, language models are akin to architects designing intricate structures. However, they face a significant challenge: the data bottleneck. As we push the boundaries of computational power, the availability of fresh, human-written text becomes a limiting factor. Imagine a scenario where we’ve read every book in the digital library, and the models ask, “What’s next?”
- Computational power is increasing faster than model creation.
- Data availability is the limiting factor for model improvement.
- Human learning involves reconstructing mental steps, unlike current AI models.
“It’s like we’re decompressing all of that information, trying to understand the background knowledge that went into producing that text.” – The Deep Dive
To address this, researchers propose a novel approach called “Reasoning to Learn from Latent Thoughts.” This involves inferring the hidden thought processes that lead to the text, much like reconstructing the steps behind a finished drawing.
Unveiling Synthetic Latent Thoughts
The concept of synthetic latent thoughts revolves around using advanced language models to infer the hidden reasoning behind a piece of text. For instance, consider a mathematical proof with a conclusion stating, “therefore, X equals Y.” The synthetic latent thoughts provide background knowledge, definitions, and logical steps leading to this conclusion, even if not explicitly stated in the original text.
- Use advanced models to infer hidden reasoning.
- Synthetic latent thoughts fill in the gaps, providing context.
- Enhances understanding beyond the surface level.
This technique allows language models to learn more efficiently with limited data, as demonstrated by experiments in data-constrained settings. For example, a small language model trained with augmented data outperformed those trained on larger datasets.
Revolutionizing AI with Bootstrapping Latent Thoughts
Bootstrapping Latent Thoughts (BOLT) is an innovative iterative process that enhances model learning through self-improvement. By training a model on data with synthetic latent thoughts, and then using that model to generate even better thoughts, we create a cycle of continuous refinement.
- Train model with initial synthetic latent thoughts.
- Generate refined thoughts from the trained model.
- Use refined thoughts for further training.
Figures 8 and 11 in the research paper illustrate the significant performance gains achieved through BOLT. This approach unlocks the potential of existing data, reducing the need for continuous influxes of new information.
In the realm of video games, this technique could revolutionize NPC (non-player character) behavior. By training NPCs with latent thoughts, they could react more intelligently and realistically to player actions, creating more immersive gaming experiences.
“Imagine an NPC that witnesses a player stealing something. If its underlying model has been trained with latent thoughts about cause and effect, it might react in a way that’s much more believable.” – The Deep Dive
Conclusion: Embracing Hidden Wisdom
Our exploration into the world of latent thoughts uncovers vast potential for enhancing both AI and human understanding. By modeling hidden thinking, AI can achieve more with less data, paving the way for more efficient language models and lifelike AI in various applications. For tech enthusiasts and individuals alike, recognizing and embracing latent thoughts could enhance personal learning and problem-solving.
As we continue to unlock hidden wisdom, we invite you to consider how this concept might apply to your own experiences. Could uncovering your latent thoughts improve your understanding and reasoning? Join us next time on The Deep Dive as we explore more intriguing topics.
Thank you for joining us on this journey. We hope you found it as fascinating as we did, and we look forward to diving into new realms of knowledge with you in the future.
Leave a Reply