Summary: Building a low-latency, multi-language automatic speech recognition (ASR) service for your home network is an exciting venture that leverages powerful AI speech models for real-time transcription. This project focuses on making complex AI technology accessible and practical for home use, allowing live transcriptions powered locally. At the core of modern ASR systems are deep learning techniques, renowned for their effectiveness in handling speech recognition tasks. To streamline the deployment process, utilizing Docker can significantly enhance efficiency, enabling the transcription service to operate seamlessly on your home network. A crucial consideration is determining the specific languages your ASR service needs to support, as this will influence the choice of Whisper model size and the balance between accuracy and speed based on your hardware capabilities. By finding the optimal configuration for your needs, you can harness cutting-edge technology to create a robust, real-time transcription service tailored to your unique requirements.
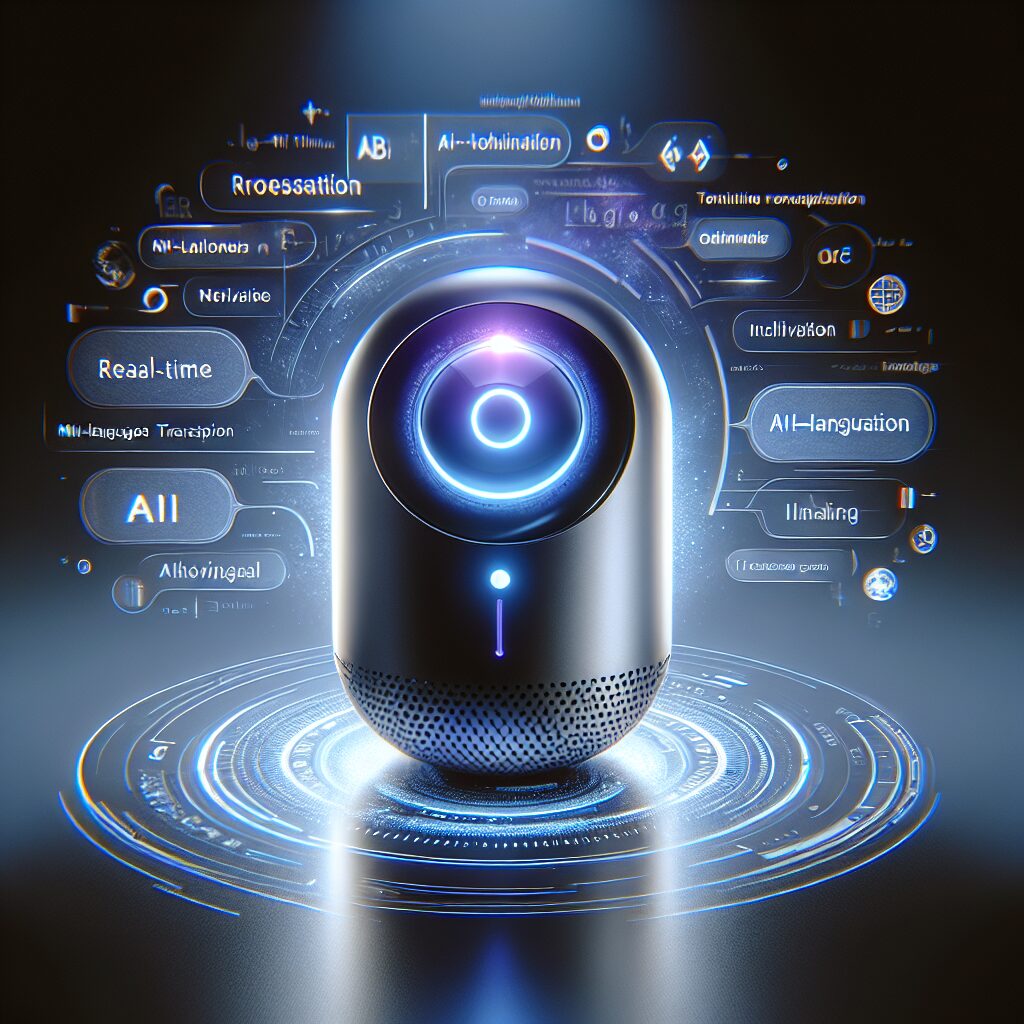
Building a low-latency, multi-language automatic speech recognition (ASR) service for your home network is an exciting venture that leverages powerful AI speech models for real-time transcription. This project focuses on making complex AI technology accessible and practical for home use, allowing live transcriptions powered locally. At the core of modern ASR systems are deep learning techniques, renowned for their effectiveness in handling speech recognition tasks. Let’s explore how you can create a robust, real-time transcription service tailored to your unique requirements.
The Magic of Deep Learning Techniques
At the heart of modern ASR systems lies deep learning—an approach that has revolutionized how machines understand human language. These techniques are incredibly powerful but also require significant computing resources, especially when aiming for real-time results.
- Moonshine: Designed for live processing, Moonshine uses rotary position embedding to enhance efficiency.
- OpenAI’s Whisper: Known for its ability to understand over 100 languages, Whisper is a leading choice for multi-language support.
- Faster Whisper: An optimized version that offers significantly reduced latency, crucial for real-time applications.
“Faster Whisper is built on C Translate 2, making it up to four times faster and more memory-efficient, while still supporting a multitude of languages.”
Implementing Your ASR Service at Home
Creating an ASR service at home involves several components, each playing a critical role in ensuring smooth operation and low latency. The following steps outline a streamlined setup:
- Backend Setup: Python is the preferred language, with frameworks like FastAPI offering asynchronous processing capabilities.
- Audio Handling: Tools like Voice Activity Detection (VAD) can ignore silent periods, optimizing processing time.
- Communication Protocols: WebSockets provide a continuous connection for real-time audio streaming and transcription.
To capture audio from devices, libraries such as SoundDevice or PyAudio are effective for client-side operations. For web applications, JavaScript paired with WebSockets can achieve similar results.
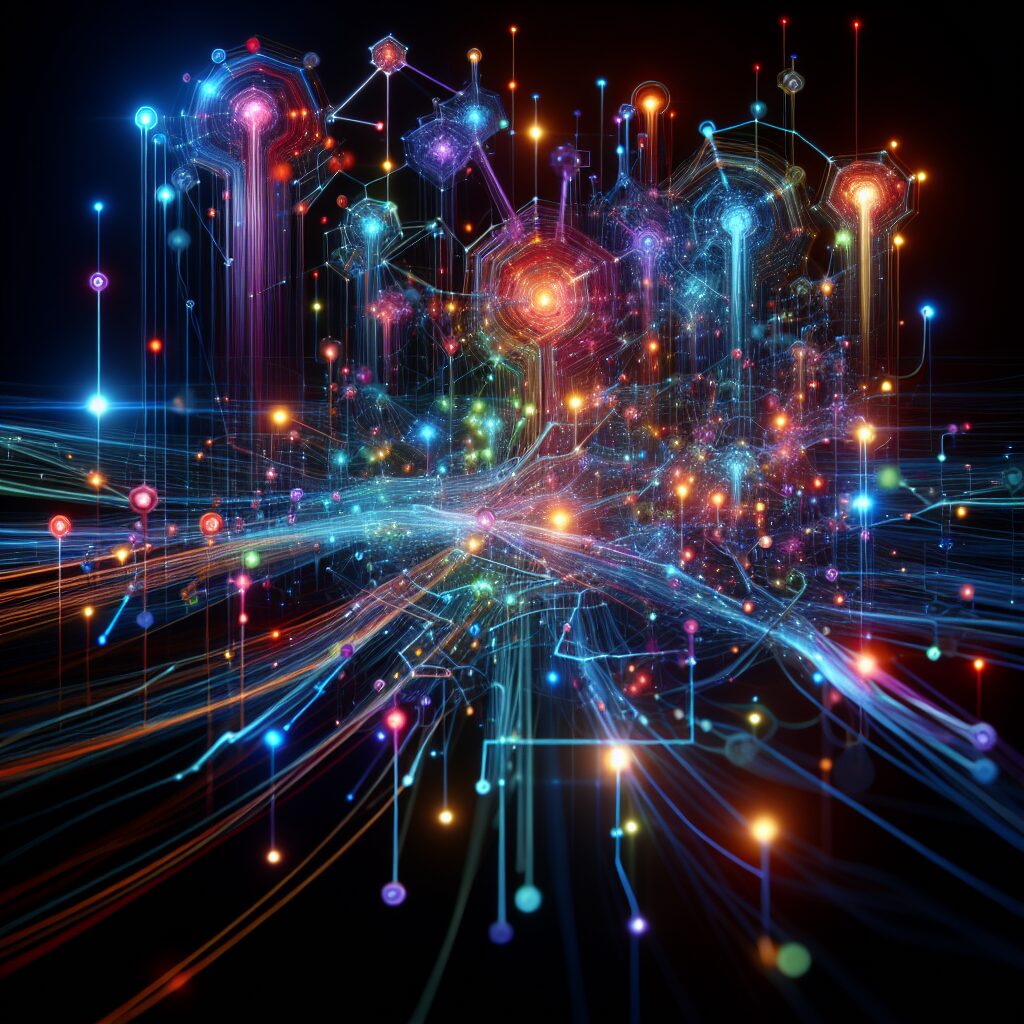
Hardware and Deployment Considerations
Choosing the right hardware is essential for achieving the desired performance in your ASR service. GPUs are particularly advantageous due to their parallel processing capabilities, which significantly reduce latency when handling AI models.
- CPU vs. GPU: While CPUs can handle the task, GPUs offer superior performance for real-time applications.
- Docker for Deployment: Docker simplifies the deployment process by packaging all necessary components into isolated environments.
- GPU Passthrough: This technique allows Docker to access your GPU, enhancing processing capabilities.
For a seamless deployment, consider using existing Docker images of Faster Whisper and set up GPU passthrough using the NVIDIA container toolkit.
“The performance data shows a stark difference: transcribing with a GPU versus a CPU is like night and day, especially for larger models.”
When configuring your setup, consider the specific languages you need to support, as this will influence the Whisper model size and the balance between accuracy and speed based on your hardware capabilities.
Summary
Building a real-time, multi-language ASR service for your home network is a rewarding project that harnesses cutting-edge AI technology. By leveraging deep learning techniques, optimized models like Faster Whisper, and efficient deployment tools such as Docker, you can create a system that delivers fast and accurate transcriptions for a variety of languages. Remember, the key to success lies in selecting the right hardware and software combination tailored to your specific needs.
Leave a Reply