Summary: In today’s rapidly evolving technological landscape, the ability of computers to recognize and identify different speakers in audio recordings is revolutionizing how we interact with digital content. This innovative technology, known as speaker recognition and speaker identification, is becoming increasingly vital across various fields. Beyond mere transcription, it enables systems to discern who is speaking, thus unlocking deeper insights into audio data. This advancement enhances efficiency in meeting note-taking and improves accessibility in podcasts, among other applications. The technology is integrated into backend frameworks like Flask and Django, and even in game development platforms like Unity, utilizing services such as AWS Transcribe, Azure, and Google Cloud. As these systems continue to evolve, the role of large language models is anticipated to expand, further refining their capabilities. The implications are vast, prompting us to ponder the myriad potential applications and possibilities this technology can offer in the near future.
“`html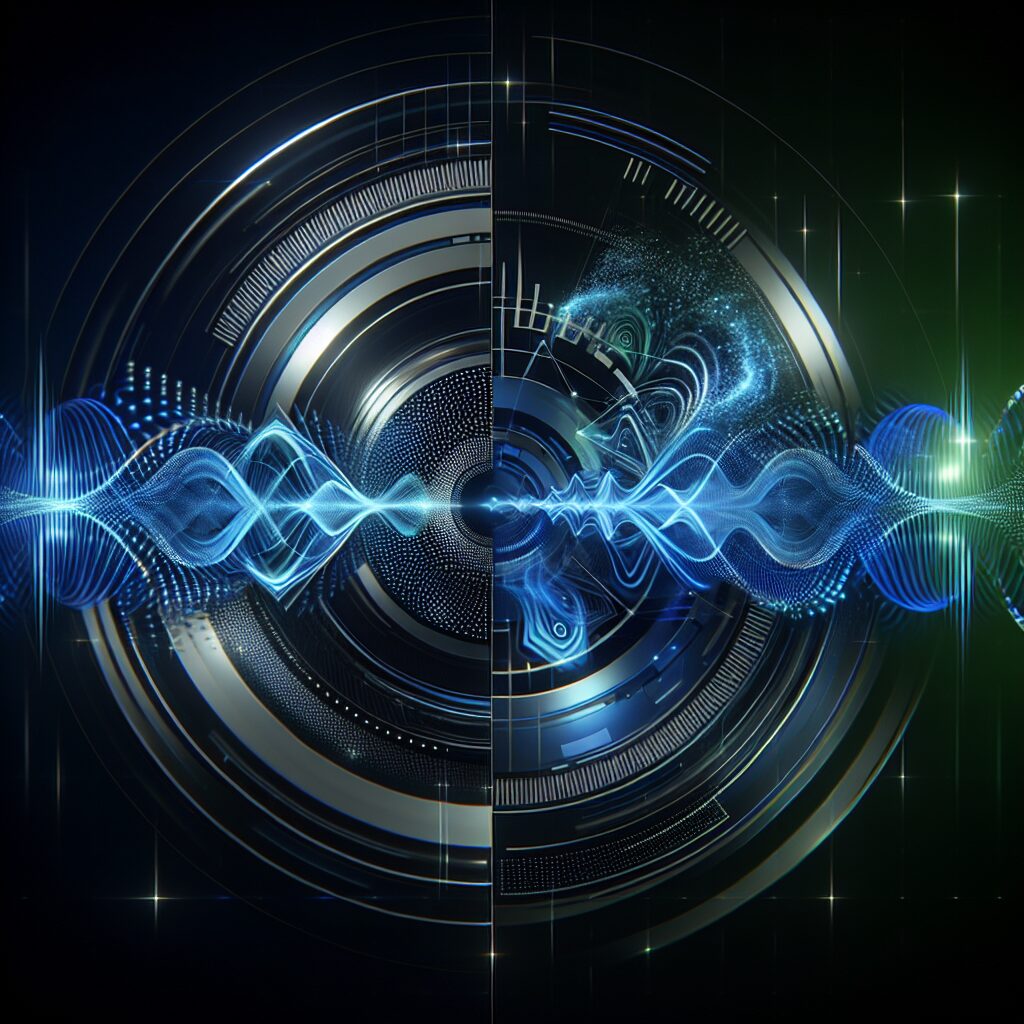
In today’s rapidly evolving technological landscape, the ability of computers to recognize and identify different speakers in audio recordings is revolutionizing how we interact with digital content. This innovative technology, known as speaker recognition and speaker identification, is becoming increasingly vital across various fields. Beyond mere transcription, it enables systems to discern who is speaking, thus unlocking deeper insights into audio data. This advancement enhances efficiency in meeting note-taking and improves accessibility in podcasts, among other applications.
Understanding the Basics: Speaker Recognition and Diarization
Before diving into the complexities, let’s clarify some basic terms that underpin this technology:
- Speaker Recognition: Verifies if a specific voice belongs to a particular person.
- Speaker Identification: Determines which person from a group is speaking.
- Speaker Diarization: Identifies who spoke when within an audio recording.
At the heart of these processes is the fact that every voice has unique characteristics, akin to a vocal fingerprint. Technologies like Feature Extraction isolate essential elements of a voice, employing methods like Mel Frequency Cepstral Coefficients (MFCCs) and advanced speaker embeddings to analyze voices beyond just the words spoken.
Technological Techniques and Their Applications
The journey from raw audio to recognized voices is intricate, involving several technological techniques:
- Text-Dependent Systems: Require a specific phrase for recognition, useful in voice authentication.
- Text-Independent Systems: Function with any speech, ideal for natural conversation transcription.
For handling multiple speakers, systems employ:
- Speaker Segmentation: Divides audio based on voice changes.
- Speaker Clustering: Groups similar voice segments, assigning labels like Speaker 1, Speaker 2, etc.
- End-to-End Neural Diarization (END): Directly outputs who is speaking and when, bypassing traditional step-by-step methods.
“Speaker recognition is not just about identifying the words, but understanding the nuances and characteristics of the speaker’s voice.” – A Voice Technology Expert
Real-World Applications and Future Directions
This technology’s applications are vast and varied, integrating into numerous tools and services:
- Call Centers: Use speaker identification to recognize returning customers.
- Live Transcriptions: Platforms like Stream and Speechly provide real-time audio processing.
- Game Development: Unity uses cloud services like AWS Transcribe for immersive experiences.
- Open-Source Tools: Libraries like Pano.audio and Speechbrain offer robust diarization capabilities.
As this technology continues to evolve, challenges remain, such as balancing speed, accuracy, and computational resources, especially for real-time applications. The natural variability of speech, including accents and emotions, adds complexity, but advancements in AI offer promising solutions.
Metrics like Diarization Error Rate (DER) and Word Error Rate (WAR) measure performance, guiding improvements in future systems. With large language models anticipated to enhance these technologies further, the potential applications are limitless, encouraging developers and researchers to explore new possibilities.
Summary
Speaker recognition and identification technologies are transforming how we interact with audio data, offering insights that go beyond simple transcription. With applications spanning from call centers to game development, the future of audio processing looks bright. As these systems become more sophisticated, they promise to unlock even more innovative uses, paving the way for a future where machines understand human speech as intricately as humans do.
What potential applications of this technology excite you the most? Share your thoughts and ideas, and let’s explore the future of audio analysis together.
“`
Leave a Reply